Image Stitching for NVIDIA Jetson/Performance/Orin: Difference between revisions
(→4K) |
|||
(21 intermediate revisions by 2 users not shown) | |||
Line 2: | Line 2: | ||
{{Image_Stitching_for_NVIDIA_Jetson/Head|previous=Performance|next=Performance/Xavier|metakeywords=Image Stitching, CUDA, Stitcher, OpenCV, Panorama}} | {{Image_Stitching_for_NVIDIA_Jetson/Head|previous=Performance|next=Performance/Xavier|metakeywords=Image Stitching, CUDA, Stitcher, OpenCV, Panorama}} | ||
</noinclude> | </noinclude> | ||
{{DISPLAYTITLE: Performance of the Stitcher element on NVIDIA Orin|noerror}} | {{DISPLAYTITLE: Performance of the Stitcher element on NVIDIA Orin|noerror}} | ||
Line 14: | Line 11: | ||
For reference, you will find in each section the homographies calibration file used for the testing. The performance results can vary depending on the homographies, but on average the following results represent the overall performance of the element. | For reference, you will find in each section the homographies calibration file used for the testing. The performance results can vary depending on the homographies, but on average the following results represent the overall performance of the element. | ||
== | == Platforms Setup == | ||
The testing for the AGX Orin was done with and without jetson clocks with the mode of 30W and JP 6.0. | |||
The Orin NX was tested with the mode of 30W and JP 5.1.2, with and without Jetson Clocks. To activate Jetson Clocks, you can do it as follows: | |||
<source lang=bash> | <source lang=bash> | ||
sudo jetson_clocks | |||
</source> | </source> | ||
= AGX Orin = | |||
== Framerate == | |||
=== 1920x1080 === | |||
The next graph shows the amount of fps for each setup of inputs with and without jetson clocks. | |||
[[File:FPS 1920X1080 AGX ORIN.png|thumb|center|1050x500px|FPS on 1920x1080 images with and without jetson_clocks.sh]] | |||
</ | === 4K === | ||
The next graph shows the amount of fps for each setup of inputs with and without jetson clocks. | |||
[[File:FPS RESULTS STITCHER 4K.png|1050x500px|thumb|center|FPS on 3840x2160 images with and without jetson_clocks.sh]] | |||
== Latency == | |||
Using the same setup as the case for framerate, for the purpose of this performance evaluation, '''Latency''' is measured as the time difference between the src of the element before the stitcher and the src of the stitcher itself, effectively measuring the time between input and output pads. For multiple inputs, the largest time difference is taken. | |||
These latency measurements were taken using the [https://developer.ridgerun.com/wiki/index.php?title=GstShark GstShark] interlatency tracer. | |||
The pictures below show the latency of the ''cuda-stitcher'' element, for multiple input images and multiple resolutions, as well as using and not using the ''jetson_clocks'' script. | |||
Using the same calibration files from the framerate performance you can achieve the following results for latency. | |||
[[File:Latencyorinagx1920stitcher.png|1000x500px|thumb|center|Latency on 1920x1080 images with and without jetson_clocks.sh]] | |||
[[File:Latency4kstitcherorinagx.png|1000x500px|thumb|center|Latency on 3840x2160 images with and without jetson_clocks.sh]] | |||
= Orin NX = | |||
== Framerate == | |||
=== 1920x1080 === | |||
The next graph shows the amount of fps for each setup of inputs with and without jetson clocks. | |||
[[File:FPS1920x1080nxorinStitcher.png|1000x500px|thumb|center|FPS on 1920x1080 images with and without jetson_clocks.sh]] | |||
=== 4K === | |||
The next graph shows the amount of fps for each setup of inputs with and without jetson clocks. | |||
[[File:FPS4kOrinNXStitcher.png|1000x500px|thumb|center|FPS on 3840x2160 images with and without jetson_clocks.sh]] | |||
== Latency == | |||
Following the same structure for latency in the AGX Orin, the pictures below show the latency of the ''cuda-stitcher'' element, for multiple input images and multiple resolutions, as well as using and not using the ''jetson_clocks'' script. | |||
[[File:Latencystitcherorinnx.png|1000x500px|thumb|center|Latency on 1920x1080 images with and without jetson_clocks.sh]] | |||
[[File:Latencystitcherorinnx4k.png|1000x500px|thumb|center|Latency on 3840x2160 images with and without jetson_clocks.sh]] | |||
= Jetson Orin Platforms CPU Usage = | |||
In the following table, you can see the performance with and without Jetson Clocks for different platforms from the Orin family with cases of 2 and 6 input video sources with a resolution of 1920x1080 with 60fps. | |||
<center> | |||
{| class="wikitable" | |||
|+ CPU Usage percentage for each platform | |||
|- | |||
! rowspan=2|Platform !! rowspan=2|Mode !! rowspan=2|Cameras !! colspan="13"| CPU | |||
|- | |||
! Avg !! 1 !! 2 !! 3 !! 4 !! 5 !! 6 !! 7 !! 8 !! 9 !! 10 !! 11 !! 12 | |||
|- | |||
| rowspan=4|Orin NX || rowspan=2|Normal || 2 || 14% || 10% || 31% || 22% || 11% || 7% || 13% || 11% || 6% || - || - || - || - | |||
|- | |||
| 6 || 7% || 15% || 13% || 14% || 13% || 0% || 0% || 0% || 0% || - || - || - || - | |||
|- | |||
| rowspan=2|Jetson Clocks || 2 || 11% || 5% || 6% || 24% || 15% || 18% || 1% || 10% || 8% || - || - || - || - | |||
|- | |||
| 6 || 5% || 8% || 9% || 8% || 7% || 1% || 1% || 4% || 3% || - || - || - || - | |||
|- | |||
| rowspan=4|AGX Orin || rowspan=2|Normal || 2 || 16% || 17% || 12% || 9% || 8% || 19% || 7% || 29% || 28% || - || - || - || - | |||
|- | |||
| 6 || 13% || 19% || 11% || 8% || 8% || 16% || 15% || 11% || 12% || - || - || - || - | |||
|- | |||
| rowspan=2|Jetson Clocks || 2 || 17% || 13% || 21% || 3% || 1% || 29% || 29% || 22% || 17% || - || - || - || - | |||
|- | |||
| 6 || 9% || 13% || 14% || 9% || 8% || 7% || 7% || 6% || 5% || - || - || - || - | |||
|} | |||
</center> | |||
The values of CPU for 6 cameras are lower because of the hight performance of the GPU affecting in lower framerate. A lower framerate implies, lower CPU consumption. | |||
= Jetson Orin Platforms GPU usage = | |||
In the following table, you can see the performance with and without Jetson Clocks for different platforms from the Orin family with cases of 2 and 6 input video sources with a resolution of 1920x1080 with 60fps. | |||
<center> | |||
{| class="wikitable" | |||
|+ GPU and RAM Usage percentage for each platform | |||
|- | |||
! Platform !! Mode !! Cameras !! GPU || RAM | |||
|- | |||
| rowspan=4|Orin Nx || rowspan=2|Normal || 2 || 66%.13 || 9.09% | |||
|- | |||
| 6 || 85.67% || 9.14% | |||
|- | |||
| rowspan=2|Jetson Clocks || 2 || 72.6% || 8.11% | |||
|- | |||
| 6 || 87.07% || 9.36 | |||
|- | |||
| rowspan=4|Orin AGX || rowspan=2|Normal || 2 || 58.63% || 5.77% | |||
|- | |||
| 6 || 76.16% || 6.77% | |||
|- | |||
| rowspan=2|Jetson Clocks || 2 || 59.32% || 6.668% | |||
|- | |||
| 6 || 81.68% || 6.82% | |||
|} | |||
</center> | |||
= Reproducing the Results = | |||
Following you can find the files and pipelines used for the test of performance. | |||
== Calibration Files | == Calibration Files == | ||
=== 1920x1080 === | === 1920x1080 === | ||
In the case of 1920x1080 resolution, for 2, 3, and 6 inputs the homographies files are the following: | In the case of 1920x1080 resolution, for 2, 3, and 6 inputs the homographies files are the following: | ||
<div class="toccolours mw-collapsible mw-collapsed" style="width:600px;"> | |||
<div style="font-weight:bold;line-height:2.6;">2 Inputs</div> | |||
<div class="mw-collapsible-content"> | |||
<source lang=bash> | <source lang=bash> | ||
Line 87: | Line 173: | ||
} | } | ||
</source> | </source> | ||
</div></div> | |||
<div class="toccolours mw-collapsible mw-collapsed" style="width:600px;"> | |||
<div style="font-weight:bold;line-height:2.6;">3 Inputs</div> | |||
<div class="mw-collapsible-content"> | |||
<source lang=bash> | <source lang=bash> | ||
{ | { | ||
Line 129: | Line 218: | ||
} | } | ||
</source> | </source> | ||
</div></div> | |||
<div class="toccolours mw-collapsible mw-collapsed" style="width:600px; overflow:auto;"> | |||
<div style="font-weight:bold;line-height:2.6;">6 Inputs</div> | |||
<div class="mw-collapsible-content"> | |||
<source lang=bash> | <source lang=bash> | ||
{ | { | ||
Line 221: | Line 314: | ||
} | } | ||
</source> | </source> | ||
</div></div> | |||
=== 4k === | === 4k === | ||
In the case of 4K resolution, for | In the case of 4K resolution, for 2,3, and 6 inputs the homographies files are the following: | ||
<div class="toccolours mw-collapsible mw-collapsed" style="width:600px; overflow:auto;"> | |||
<div style="font-weight:bold;line-height:2.6;">2 Inputs</div> | |||
<div class="mw-collapsible-content"> | |||
<source lang=bash> | <source lang=bash> | ||
{ | { | ||
Line 249: | Line 344: | ||
} | } | ||
</source> | </source> | ||
</div></div> | |||
<div class="toccolours mw-collapsible mw-collapsed" style="width:600px; overflow:auto;"> | |||
<div style="font-weight:bold;line-height:2.6;">3 Inputs</div> | |||
<div class="mw-collapsible-content"> | |||
<source lang=bash> | <source lang=bash> | ||
{ | { | ||
Line 290: | Line 389: | ||
} | } | ||
</source> | </source> | ||
</div></div> | |||
<div class="toccolours mw-collapsible mw-collapsed" style="width:600px; overflow:auto;"> | |||
<div style="font-weight:bold;line-height:2.6;">6 Inputs</div> | |||
<div class="mw-collapsible-content"> | |||
<source lang=bash> | <source lang=bash> | ||
{ | { | ||
Line 382: | Line 485: | ||
} | } | ||
</source> | </source> | ||
</div></div> | |||
= | == Pipeline structure == | ||
To replicate the results using your images, videos, or cameras, you can use the following pipeline as a base for the case of 2 cameras, then you can add the other inputs for the other cases. Also, you can adjust the resolution if needed. | |||
<source lang=bash> | |||
INPUT_0=<VIDEO_INPUT_0> | |||
INPUT_1=<VIDEO_INPUT_1> | |||
gst-launch-1.0 -e cudastitcher name=stitcher \ | |||
homography-list="`cat homographies.json | tr -d "\n" | tr -d " "`" \ | |||
filesrc location=$INPUT_0 ! qtdemux ! h264parse ! nvv4l2decoder ! queue ! nvvidconv ! stitcher.sink_0 \ | |||
filesrc location=$INPUT_1 ! qtdemux ! h264parse ! nvv4l2decoder ! queue ! nvvidconv ! stitcher.sink_1 \ | |||
stitcher. ! perf print-cpu-load=true ! fakesink -v | |||
</source> | |||
<source lang=bash> | <source lang=bash> | ||
INPUT_0=<VIDEO_INPUT_0> | |||
INPUT_1=<VIDEO_INPUT_1> | |||
INPUT_2=<VIDEO_INPUT_2> | |||
gst-launch-1.0 -e cudastitcher name=stitcher \ | |||
homography-list="`cat homographies.json | tr -d "\n" | tr -d " "`" \ | |||
filesrc location=$INPUT_0 ! qtdemux ! h264parse ! nvv4l2decoder ! queue ! nvvidconv ! stitcher.sink_0 \ | |||
filesrc location=$INPUT_1 ! qtdemux ! h264parse ! nvv4l2decoder ! queue ! nvvidconv ! stitcher.sink_1 \ | |||
filesrc location=$INPUT_2 ! qtdemux ! h264parse ! nvv4l2decoder ! queue ! nvvidconv ! stitcher.sink_2 \ | |||
stitcher. ! perf print-cpu-load=true ! fakesink -v | |||
</source> | </source> | ||
= | <source lang=bash> | ||
INPUT_0=<VIDEO_INPUT_0> | |||
= | INPUT_1=<VIDEO_INPUT_1> | ||
INPUT_2=<VIDEO_INPUT_2> | |||
INPUT_3=<VIDEO_INPUT_3> | |||
INPUT_4=<VIDEO_INPUT_4> | |||
INPUT_5=<VIDEO_INPUT_5> | |||
gst-launch-1.0 -e cudastitcher name=stitcher \ | |||
= | homography-list="`cat homographies.json | tr -d "\n" | tr -d " "`" \ | ||
filesrc location=$INPUT_0 ! qtdemux ! h264parse ! nvv4l2decoder ! queue ! nvvidconv ! stitcher.sink_0 \ | |||
filesrc location=$INPUT_1 ! qtdemux ! h264parse ! nvv4l2decoder ! queue ! nvvidconv ! stitcher.sink_1 \ | |||
filesrc location=$INPUT_2 ! qtdemux ! h264parse ! nvv4l2decoder ! queue ! nvvidconv ! stitcher.sink_2 \ | |||
filesrc location=$INPUT_3 ! qtdemux ! h264parse ! nvv4l2decoder ! queue ! nvvidconv ! stitcher.sink_3 \ | |||
filesrc location=$INPUT_4 ! qtdemux ! h264parse ! nvv4l2decoder ! queue ! nvvidconv ! stitcher.sink_4 \ | |||
= | filesrc location=$INPUT_5 ! qtdemux ! h264parse ! nvv4l2decoder ! queue ! nvvidconv ! stitcher.sink_5 \ | ||
stitcher. ! perf print-cpu-load=true ! fakesink -v | |||
</source> | |||
= | |||
= | |||
= | |||
|- | |||
! | |||
</ | |||
< | <noinclude> | ||
{| | {{Image_Stitching_for_NVIDIA_Jetson/Foot|Performance|Performance/Xavier}} | ||
</noinclude> | |||
|} | |||
</ |
Latest revision as of 20:40, 19 September 2024
Image Stitching for NVIDIA®Jetson™ |
---|
![]() |
Before Starting |
Image Stitching Basics |
Overview |
Getting Started |
User Guide |
Resources |
Examples |
Spherical Video |
Performance |
Contact Us |
![]() |
The performance of the cuda stitcher element depends on many factors, being more significant than those that have a direct influence on the output resolution.
The following sections show the measurements of the cuda-stitcher (FPS and Latency) for multiple image resolutions; as well as the impact of changing parameters such as the blending width and the homography-list.
For reference, you will find in each section the homographies calibration file used for the testing. The performance results can vary depending on the homographies, but on average the following results represent the overall performance of the element.
Platforms Setup
The testing for the AGX Orin was done with and without jetson clocks with the mode of 30W and JP 6.0.
The Orin NX was tested with the mode of 30W and JP 5.1.2, with and without Jetson Clocks. To activate Jetson Clocks, you can do it as follows:
sudo jetson_clocks
AGX Orin
Framerate
1920x1080
The next graph shows the amount of fps for each setup of inputs with and without jetson clocks.
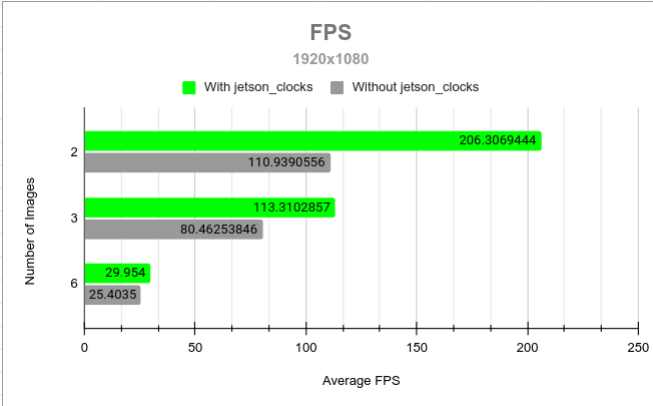
4K
The next graph shows the amount of fps for each setup of inputs with and without jetson clocks.

Latency
Using the same setup as the case for framerate, for the purpose of this performance evaluation, Latency is measured as the time difference between the src of the element before the stitcher and the src of the stitcher itself, effectively measuring the time between input and output pads. For multiple inputs, the largest time difference is taken.
These latency measurements were taken using the GstShark interlatency tracer.
The pictures below show the latency of the cuda-stitcher element, for multiple input images and multiple resolutions, as well as using and not using the jetson_clocks script.
Using the same calibration files from the framerate performance you can achieve the following results for latency.


Orin NX
Framerate
1920x1080
The next graph shows the amount of fps for each setup of inputs with and without jetson clocks.

4K
The next graph shows the amount of fps for each setup of inputs with and without jetson clocks.

Latency
Following the same structure for latency in the AGX Orin, the pictures below show the latency of the cuda-stitcher element, for multiple input images and multiple resolutions, as well as using and not using the jetson_clocks script.


Jetson Orin Platforms CPU Usage
In the following table, you can see the performance with and without Jetson Clocks for different platforms from the Orin family with cases of 2 and 6 input video sources with a resolution of 1920x1080 with 60fps.
Platform | Mode | Cameras | CPU | ||||||||||||
---|---|---|---|---|---|---|---|---|---|---|---|---|---|---|---|
Avg | 1 | 2 | 3 | 4 | 5 | 6 | 7 | 8 | 9 | 10 | 11 | 12 | |||
Orin NX | Normal | 2 | 14% | 10% | 31% | 22% | 11% | 7% | 13% | 11% | 6% | - | - | - | - |
6 | 7% | 15% | 13% | 14% | 13% | 0% | 0% | 0% | 0% | - | - | - | - | ||
Jetson Clocks | 2 | 11% | 5% | 6% | 24% | 15% | 18% | 1% | 10% | 8% | - | - | - | - | |
6 | 5% | 8% | 9% | 8% | 7% | 1% | 1% | 4% | 3% | - | - | - | - | ||
AGX Orin | Normal | 2 | 16% | 17% | 12% | 9% | 8% | 19% | 7% | 29% | 28% | - | - | - | - |
6 | 13% | 19% | 11% | 8% | 8% | 16% | 15% | 11% | 12% | - | - | - | - | ||
Jetson Clocks | 2 | 17% | 13% | 21% | 3% | 1% | 29% | 29% | 22% | 17% | - | - | - | - | |
6 | 9% | 13% | 14% | 9% | 8% | 7% | 7% | 6% | 5% | - | - | - | - |
The values of CPU for 6 cameras are lower because of the hight performance of the GPU affecting in lower framerate. A lower framerate implies, lower CPU consumption.
Jetson Orin Platforms GPU usage
In the following table, you can see the performance with and without Jetson Clocks for different platforms from the Orin family with cases of 2 and 6 input video sources with a resolution of 1920x1080 with 60fps.
Platform | Mode | Cameras | GPU | RAM |
---|---|---|---|---|
Orin Nx | Normal | 2 | 66%.13 | 9.09% |
6 | 85.67% | 9.14% | ||
Jetson Clocks | 2 | 72.6% | 8.11% | |
6 | 87.07% | 9.36 | ||
Orin AGX | Normal | 2 | 58.63% | 5.77% |
6 | 76.16% | 6.77% | ||
Jetson Clocks | 2 | 59.32% | 6.668% | |
6 | 81.68% | 6.82% |
Reproducing the Results
Following you can find the files and pipelines used for the test of performance.
Calibration Files
1920x1080
In the case of 1920x1080 resolution, for 2, 3, and 6 inputs the homographies files are the following:
{ "homographies": [ { "images": { "target": 1, "reference": 0 }, "matrix": { "h00": 0.7490261895239074, "h01": 0.04467113632580552, "h02": 1018.9828151317821, "h10": -0.05577820485200396, "h11": 0.9590844935041531, "h12": 61.08068248533324, "h20": -0.00014412069693060743, "h21": 1.7581178118418628e-05, "h22": 1.0 } } ] }
{ "homographies": [ { "images": { "target": 1, "reference": 0 }, "matrix": { "h00": 0.7490261895239074, "h01": 0.04467113632580552, "h02": 1018.9828151317821, "h10": -0.05577820485200396, "h11": 0.9590844935041531, "h12": 61.08068248533324, "h20": -0.00014412069693060743, "h21": 1.7581178118418628e-05, "h22": 1.0 } }, { "images": { "target": 2, "reference": 0 }, "matrix": { "h00": 1.3197060186315637, "h01": -0.10518566348433173, "h02": -1264.5768270277113, "h10": 0.1467783274677278, "h11": 1.1524649023229194, "h12": -227.0179395401691, "h20": 0.00019864314625771476, "h21": -0.00010857278904972765, "h22": 1.0 } } ] }
{ "homographies": [ { "images": { "target": 1, "reference": 0 }, "matrix": { "h00": 0.7490261895239074, "h01": 0.04467113632580552, "h02": 1018.9828151317821, "h10": -0.05577820485200396, "h11": 0.9590844935041531, "h12": 61.08068248533324, "h20": -0.00014412069693060743, "h21": 1.7581178118418628e-05, "h22": 1.0 } }, { "images": { "target": 2, "reference": 0 }, "matrix": { "h00": 0.8203959419915308, "h01": 0.19134092629013782, "h02": 927.0948457177544, "h10": -0.0179915273643625, "h11": 1.034698464498257, "h12": -680.8085473782533, "h20": -0.00014834561743950648, "h21": 8.652704821052748e-05, "h22": 1.0 } }, { "images": { "target": 3, "reference": 0 }, "matrix": { "h00": 1.0378054016500433, "h01": 0.03676895233913665, "h02": -5.558535987201656, "h10": -0.006201947768703567, "h11": 1.0395215780726415, "h12": -621.329917462604, "h20": -1.2684476455313587e-05, "h21": 5.7273947504607006e-05, "h22": 1.0 } }, { "images": { "target": 4, "reference": 0 }, "matrix": { "h00": 1.3394576098442972, "h01": -0.15576318123486257, "h02": -1230.4161628261922, "h10": 0.21296156399303987, "h11": 1.25689163996496, "h12": -1130.7835925176462, "h20": 0.00014172905668812799, "h21": 4.9304913992162066e-05, "h22": 1.0 } }, { "images": { "target": 5, "reference": 0 }, "matrix": { "h00": 1.3197060186315637, "h01": -0.10518566348433173, "h02": -1264.5768270277113, "h10": 0.1467783274677278, "h11": 1.1524649023229194, "h12": -227.0179395401691, "h20": 0.00019864314625771476, "h21": -0.00010857278904972765, "h22": 1.0 } } ] }
4k
In the case of 4K resolution, for 2,3, and 6 inputs the homographies files are the following:
{ "homographies": [ { "images": { "target": 1, "reference": 0 }, "matrix": { "h00": 0.7014208032457997, "h01": 0.0, "h02": 3180.223613728557, "h10": -0.044936941010614385, "h11": 0.9201121048700188, "h12": 86.2789267403796, "h20": -4.160827871353184e-05, "h21": 0.0, "h22": 1.0 } } ] }
{ "homographies": [ { "images": { "target": 1, "reference": 0 }, "matrix": { "h00": 0.7014208032457997, "h01": 0.0, "h02": 3180.223613728557, "h10": -0.044936941010614385, "h11": 0.9201121048700188, "h12": 86.2789267403796, "h20": -4.160827871353184e-05, "h21": 0.0, "h22": 1.0 } }, { "images": { "target": 2, "reference": 0 }, "matrix": { "h00": 1.0059596024427553, "h01": 0.0, "h02": -3065.535683195869, "h10": 0.02812139261826276, "h11": 1.0500863393572026, "h12": -56.09324650577886, "h20": 2.60866350818764e-05, "h21": 0.0, "h22": 1.0 } } ] }
{ "homographies": [ { "images": { "target": 1, "reference": 0 }, "matrix": { "h00": 1.069728304504107, "h01": 0.003976349281680208, "h02": 2486.8360288823615, "h10": 0.04509436469518921, "h11": 1.036853809177049, "h12": -90.69673725525423, "h20": 9.660223055111373e-06, "h21": 9.013807989685773e-06, "h22": 1.0 } }, { "images": { "target": 2, "reference": 0 }, "matrix": { "h00": 1.1029118727474485, "h01": 0.043952634510905024, "h02": 2444.964028928727, "h10": 0.024629835881337415, "h11": 1.0529792960117825, "h12": -146.73010297262417, "h20": 1.4490325579825004e-05, "h21": 1.404662537183237e-05, "h22": 1.0 } }, { "images": { "target": 3, "reference": 0 }, "matrix": { "h00": 0.8848587011291174, "h01": -0.20469564243712468, "h02": 221.0712938320948, "h10": 0.0, "h11": 0.3625879945792258, "h12": 1319.7216938381491, "h20": 0.0, "h21": -0.00010661231376933578, "h22": 1.0 } }, { "images": { "target": 4, "reference": 0 }, "matrix": { "h00": 0.8892810916856179, "h01": -0.4749636131671599, "h02": 2925.5803039636144, "h10": 0.0, "h11": 0.37335000737513163, "h12": 1316.6516233548427, "h20": 0.0, "h21": -0.00010251750769850202, "h22": 1.0 } }, { "images": { "target": 5, "reference": 0 }, "matrix": { "h00": 1.004086737535137, "h01": -0.012649881236723413, "h02": 9.93271028892309, "h10": 0.003748731449662241, "h11": 0.9950228623682942, "h12": -4.0743393651017925, "h20": 1.6341487799222761e-06, "h21": -3.0483164302875094e-06, "h22": 1.0 } } ] }
Pipeline structure
To replicate the results using your images, videos, or cameras, you can use the following pipeline as a base for the case of 2 cameras, then you can add the other inputs for the other cases. Also, you can adjust the resolution if needed.
INPUT_0=<VIDEO_INPUT_0> INPUT_1=<VIDEO_INPUT_1> gst-launch-1.0 -e cudastitcher name=stitcher \ homography-list="`cat homographies.json | tr -d "\n" | tr -d " "`" \ filesrc location=$INPUT_0 ! qtdemux ! h264parse ! nvv4l2decoder ! queue ! nvvidconv ! stitcher.sink_0 \ filesrc location=$INPUT_1 ! qtdemux ! h264parse ! nvv4l2decoder ! queue ! nvvidconv ! stitcher.sink_1 \ stitcher. ! perf print-cpu-load=true ! fakesink -v
INPUT_0=<VIDEO_INPUT_0> INPUT_1=<VIDEO_INPUT_1> INPUT_2=<VIDEO_INPUT_2> gst-launch-1.0 -e cudastitcher name=stitcher \ homography-list="`cat homographies.json | tr -d "\n" | tr -d " "`" \ filesrc location=$INPUT_0 ! qtdemux ! h264parse ! nvv4l2decoder ! queue ! nvvidconv ! stitcher.sink_0 \ filesrc location=$INPUT_1 ! qtdemux ! h264parse ! nvv4l2decoder ! queue ! nvvidconv ! stitcher.sink_1 \ filesrc location=$INPUT_2 ! qtdemux ! h264parse ! nvv4l2decoder ! queue ! nvvidconv ! stitcher.sink_2 \ stitcher. ! perf print-cpu-load=true ! fakesink -v
INPUT_0=<VIDEO_INPUT_0> INPUT_1=<VIDEO_INPUT_1> INPUT_2=<VIDEO_INPUT_2> INPUT_3=<VIDEO_INPUT_3> INPUT_4=<VIDEO_INPUT_4> INPUT_5=<VIDEO_INPUT_5> gst-launch-1.0 -e cudastitcher name=stitcher \ homography-list="`cat homographies.json | tr -d "\n" | tr -d " "`" \ filesrc location=$INPUT_0 ! qtdemux ! h264parse ! nvv4l2decoder ! queue ! nvvidconv ! stitcher.sink_0 \ filesrc location=$INPUT_1 ! qtdemux ! h264parse ! nvv4l2decoder ! queue ! nvvidconv ! stitcher.sink_1 \ filesrc location=$INPUT_2 ! qtdemux ! h264parse ! nvv4l2decoder ! queue ! nvvidconv ! stitcher.sink_2 \ filesrc location=$INPUT_3 ! qtdemux ! h264parse ! nvv4l2decoder ! queue ! nvvidconv ! stitcher.sink_3 \ filesrc location=$INPUT_4 ! qtdemux ! h264parse ! nvv4l2decoder ! queue ! nvvidconv ! stitcher.sink_4 \ filesrc location=$INPUT_5 ! qtdemux ! h264parse ! nvv4l2decoder ! queue ! nvvidconv ! stitcher.sink_5 \ stitcher. ! perf print-cpu-load=true ! fakesink -v