RidgeRun Detection Microservice

RidgeRun's Detection Microservice as its name indicates detects objects from an input stream. The target objects to detect are queried and described in a text prompt. The text queries will be given via API calls. And finally the service sent the detections over REDIS.

- The service supports a single input video stream obtained from VST. By default the first stream available will be used but the user can select an specific stream through the server using an API source request.
- The microservice uses the NanoOwl generative AI model for the detection. This model allows open vocabulary detection, meaning that the user can provide a list of objects that are not bounded to any specific classes. The objects to detect can be defined through the server using an API search request, with the list of objects and corresponding thresholds.
- As an output the service generates metadata with the detected object bounding boxes and classes. The metadata is sent in a Metropolis Minimal Schema through a Redis Stream called detection.
Large Images Detection
If your use case involves video images of high resolution and great differences between width and height, like 360 video footage with typical resolutions of 3840x1920 or 2160x1080, some of the objects may be too small for the detection, since the model scales the input video as part of the image preprocessing stage to a square image of 768.
If the objects are at least one third of the height of the image the detection will work just fine but to detect smaller objects the detection microservice incorporated SAHI option. SAHI alternative allows performing inference over smaller slices of the original image to detect these small objects. If enabled the application will split the image in a overlaping grid of image slices,and detect objects for each slice, the predictions of the slices are merged to provide the final bounding boxes. As you can foresee with SAHI the processing is slower because the inference will be run more than once.
By default sahi is disabled and the whole image is used at once, but it can be enabled by setting the vertical and horizontal slices arguments.

Running the Service
Launch Platform Services
This microservice requires of NVIDIA's VST and Redis Platform Services. Use systemctl to launch each of the services.
- Redis:
sudo systemctl start jetson-ingress
- VST:
sudo systemctl start jetson-vst
Using Docker
You can obtain or build a docker image for the detection microservice, check below the method of your preference. The image include a base genai image and the dependencies to run the detection microservice application. The image was developed and testing for NVIDIA JetPack 6.0. Once you get your image with either method proceed to Launch the container
Pre-build Image
You can obtain a pre-build image of the detection service from Docker Hub:
docker pull ridgerun/detection-service
Build Image
You can build the detection microservice image using the Dockerfile in the docker directory. First we need to prepare the context directory for this build, you need to create a directory and include this repository and the rrms-utils project. The Dockerfile will look for both packages in the context directory and copy them to the container.
mkdir detection-context cd detection-context git clone https://github.com/RidgeRun/detection-service.git git clone https://github.com/RidgeRun/rrms-utils.git
After this, your context directory should look like this:
detection-context/ ├── detection └── rrms-utils
Then build the container image with the following command:
DOCKER_BUILDKIT=0 docker build --network=host --tag ridgerun/detection-service --file detection-context/detection/docker/Dockerfile detection-context/
Change detection-context/ to your context's path and the tag to the name you want to give to your image.
Launch the container
You can ensure the images have started successfully by running
docker image
You should get an entry showing the ridgerun/detection-service image
nvidia@ubuntu:~$ docker images REPOSITORY TAG IMAGE ID CREATED SIZE ridgerun/detection-service latest 61342691a290 45 hours ago 18.3GB
The container can be launched by running the following command:
docker run --runtime nvidia -it --network host --name detection-service ridgerun/detection-service:latest detection --host 0.0.0.0
Here we are creating a container called detection that will start the detection application (check Using_Standalone_Application), launching the server in port 5030 and start detecting persons from the first VST stream available.
You can verify the container is running with:
docker ps
You should see an entry with the detection-service container:
CONTAINER ID IMAGE COMMAND CREATED STATUS PORTS NAMES c0245d07c273 ridgerun/detection "detection --horizon…" 11 hours ago Up 2 seconds detection-service dd200109321a nvcr.io/nvidia/jps/vst:v1.2.58_aarch64 "sh -c '/root/vst_re…" 33 hours ago Up 11 hours vst 63485ad51fe7 nvcr.io/nvidia/jps/ialpha-ingress-arm64v8:0.10 "sh -c '/nginx.sh 2>…" 45 hours ago Up 11 hours ingress 788b316fb239 redisfab/redistimeseries:master-arm64v8-jammy "docker-entrypoint.s…" 45 hours ago Up 11 hours redis
Using Standalone Application
The project is configured (via setup.py) and install the service application called detection. Just need to run:
pip install .
Then you will have the service with the following options:
usage: detection [-h] [--port PORT] [--host HOST] [--objects OBJECTS] [--thresholds THRESHOLDS] options: -h, --help show this help message and exit --port PORT Port for server --host HOST Server ip address --objects OBJECTS List of objects to detect, example: 'a person,a box,a ball' --thresholds THRESHOLDS List of thresholds corresponding to the objects, example: 0.1,0.2,0.65 --vertical-slices VERTICAL_SLICES Divide the image in given amount of vertical slices to detect small objects --horizontal-slices HORIZONTAL_SLICES Divide the image in given amount of horizontal slices to detect small objects
Notice that you can set the default detection using the objects and thresholds arguments, if not provided "a person" with a threshold of 0.2 will be used.
You just need to run the application as follows:
detection
This will start the service in address 127.0.0.0 and port 5030. If you want to serve in a different port or address, use the --port and --host options.
View Service Output
You can create a simple python application to obtain the detection output from REDIS.
First you need to install redis:
pip3 install redis
Create the application redis_detection.py with the following contents:
import redis redis_server = redis.Redis(host="localhost", port=6379, decode_responses=True) while True: l = redis_server.xread(count=10, block=5000, streams={'detection': '$'} ) print(l)
And run it:
python3 redis_detection.py
You could use the API to select which objects detect, for example with the following command you can request to detect guitars through ingress.
curl "http://<BOARD_IP>:30080/detect/search?a%20guitar&thresholds=0.2"
Using a video like the one below at the right, you will get the redis metadata as shown in the terminal, empty until a man with a guitar appear at the right of the video when metadata for detected guitar object is printed.
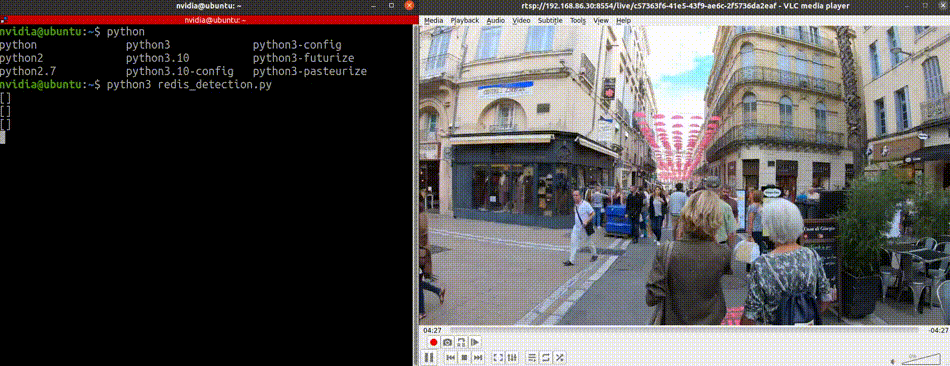